Navigating the Maze of AI Fairness: Challenges and Solutions
Key Aspects | Description |
---|---|
Definition | Systematic errors in AI systems leading to unfair outcomes |
Main Causes | Biased training data, flawed algorithms, lack of diversity in AI teams |
Impact | Discrimination in hiring, lending, criminal justice, and more |
When it comes to AI fairness, we’re dealing with a multifaceted challenge that requires a holistic approach. Consider these key points:
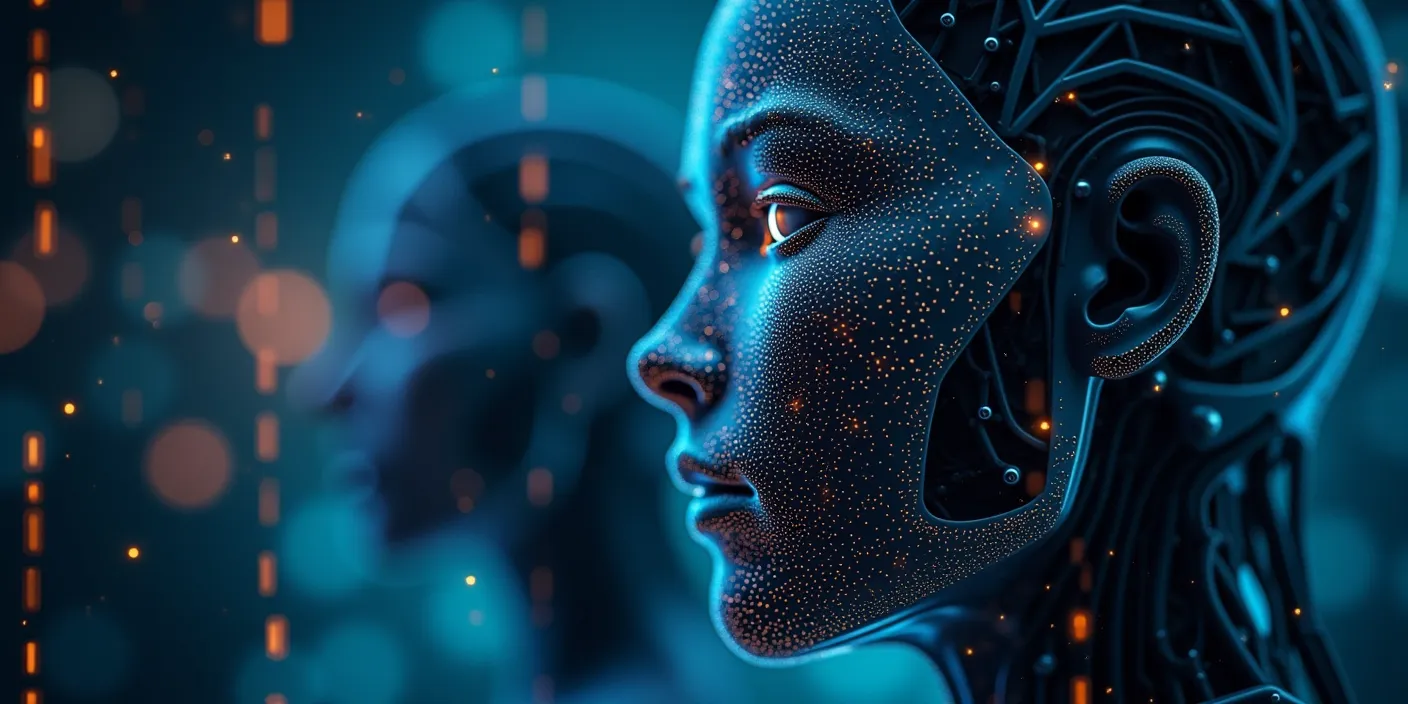
#AIFairness, #EthicalAI, #TechChallenges
- Data bias: AI systems learn from historical data, which may contain societal biases
- Algorithmic bias: The design of AI algorithms can inadvertently perpetuate or amplify existing biases
- Interpretation bias: How AI outputs are interpreted and applied can lead to unfair outcomes
“The biggest challenge in creating fair AI systems is not technical, it’s social,” says Dr. Timnit Gebru, AI ethics researcher.
FAQ: Demystifying AI Fairness
Q: What is algorithmic bias?
A: Algorithmic bias refers to systematic errors in AI systems that create unfair outcomes, often disadvantaging certain groups.
Q: How can we detect AI bias?
A: Bias can be detected through rigorous testing, diverse data sets, and ongoing monitoring of AI system outputs.
Q: Is it possible to create completely unbiased AI?
A: While perfect fairness may be unattainable, continuous efforts can significantly reduce bias in AI systems.
5 Steps to Promote AI Fairness
- Diversify AI development teams
- Use representative and balanced training data
- Implement rigorous testing for bias
- Establish clear ethical guidelines
- Maintain transparency in AI decision-making processes
According to a 2021 study by MIT, AI systems showed up to 20% higher error rates for minority groups in facial recognition tasks.
Innovative Solutions for Fair AI
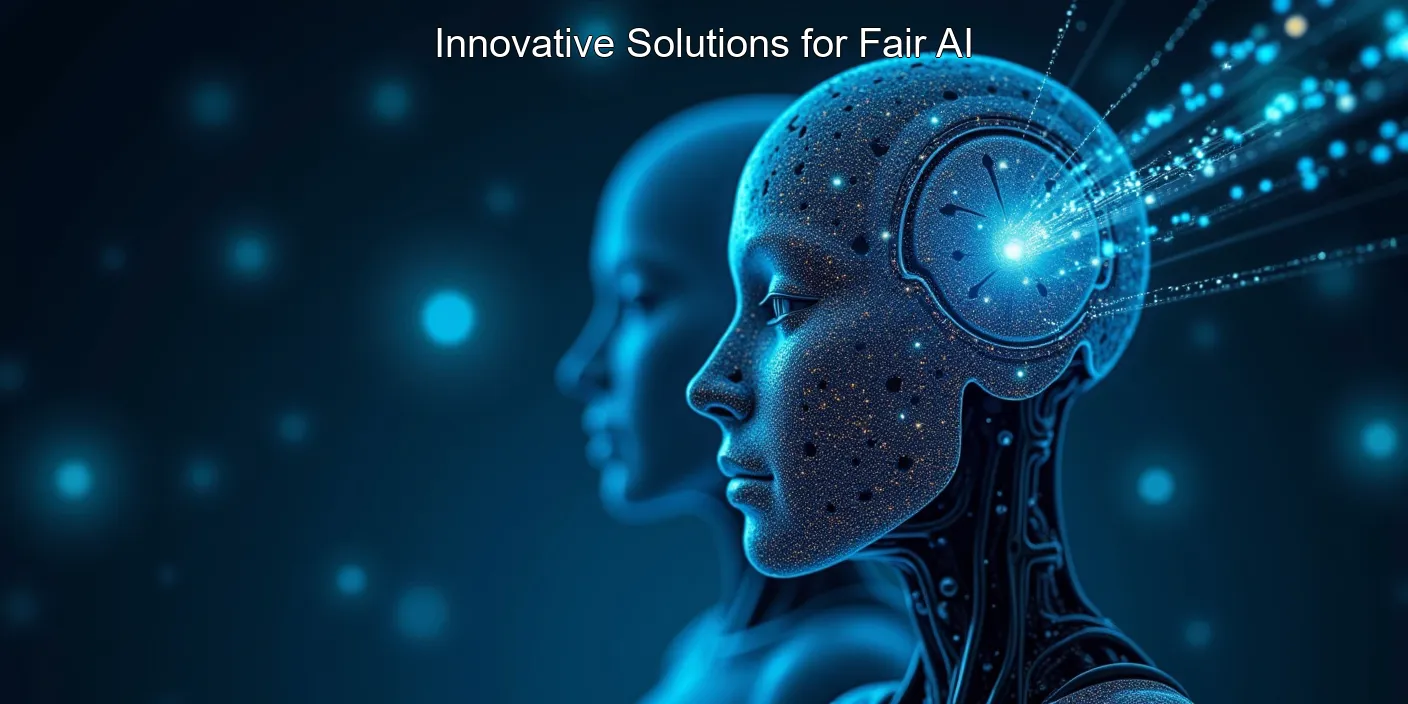
Solution | Description |
---|---|
Fairness-aware algorithms | Algorithms designed to actively mitigate bias |
Explainable AI (XAI) | Systems that provide transparent reasoning for decisions |
Bias bounties | Programs rewarding the identification of AI biases |
The quest for fair AI is driving innovation across the tech industry. Here are some groundbreaking approaches:
“Driving Fairness: Innovations in AI Tech”
- Adversarial debiasing: Using adversarial networks to remove sensitive information from data
- Federated learning: Enabling AI training on diverse, decentralized datasets
- Ethical AI frameworks: Comprehensive guidelines for developing and deploying fair AI systems
“Innovation in AI fairness isn’t just about technology—it’s about reimagining our approach to ethics in the digital age,” notes Dr. Fei-Fei Li, AI researcher and advocate.
FAQ: Exploring Fair AI Solutions
Q: What is explainable AI?
A: Explainable AI refers to systems that can provide clear, understandable reasons for their decisions and outputs.
“Demystifying Explainable AI: Clear Answers for Fair Solutions”
Q: How does federated learning promote fairness?
A: Federated learning allows AI models to be trained on diverse, decentralized data sets without compromising privacy, potentially reducing bias.
Q: Can AI be used to detect its own biases?
A: Yes, AI systems can be designed to self-monitor and flag potential biases, though human oversight remains crucial.
Best Practices for Implementing Fair AI
- Conduct regular fairness audits
- Engage diverse stakeholders in AI development
- Implement continuous monitoring and adjustment
- Prioritize transparency and accountability
- Invest in ongoing education on AI ethics
A 2022 survey by Gartner found that organizations implementing AI fairness practices saw a 35% increase in public trust and a 28% reduction in regulatory risks.
As we navigate the complex landscape of AI fairness, it’s crucial to remember that this is an ongoing journey. By combining technological innovation with ethical considerations, we can work towards AI innovations that benefit all of society. The path to fair AI intersects with ethical AI practices, shaping how AI in everyday life impacts us. Even in areas like AI in entertainment, fairness plays a crucial role in ensuring inclusive and representative experiences for all users.
Challenges in Ensuring Fairness in AI Systems: Navigating the Path to Unbiased Artificial Intelligence
As artificial intelligence continues to shape our world, ensuring fairness in AI systems has become a critical challenge. From AI in Everyday Life to complex decision-making processes, the impact of biased AI can be far-reaching. Let’s explore the hurdles and potential solutions in creating fair and unbiased AI systems.
Understanding the Fairness Dilemma in AI
Key Aspects | Challenges | Potential Solutions |
---|---|---|
Data Bias | Historical prejudices in training data | Diverse and representative datasets |
Algorithm Bias | Unintended discrimination in AI models | Fairness-aware machine learning techniques |
Transparency | Black box nature of complex AI systems | Explainable AI (XAI) approaches |
The journey towards fair AI systems is fraught with challenges:
- Identifying and mitigating hidden biases in training data
- Balancing accuracy with fairness in AI algorithms
- Ensuring transparency in AI decision-making processes
- Addressing the lack of diversity in AI development teams
“The biggest challenge in creating fair AI systems is not technical, but human. We must recognize and address our own biases to build truly unbiased AI.” – Dr. Fei-Fei Li, AI researcher and professor at Stanford University
Frequently Asked Questions about AI Fairness
Q: What is algorithmic bias in AI?
A: Algorithmic bias refers to systematic and repeatable errors in AI systems that create unfair outcomes, such as privileging one group of users over others.
Q: How can we measure fairness in AI systems?
A: Fairness can be measured using various metrics, including demographic parity, equal opportunity, and disparate impact analysis.
Q: What role does diversity play in developing fair AI?
A: Diverse development teams can help identify and mitigate potential biases, bringing different perspectives to the AI creation process.
Best Practices for Developing Fair AI Systems
- Conduct thorough bias audits of training data and algorithms
- Implement fairness constraints in machine learning models
- Increase transparency through explainable AI techniques
- Foster diverse and inclusive AI development teams
- Regularly test and monitor AI systems for fairness
According to a study by the AI Now Institute, only 18% of authors at leading AI conferences are women, and more than 80% of AI professors are men, highlighting the need for greater diversity in the field.
The Role of Ethical AI in Ensuring Fairness
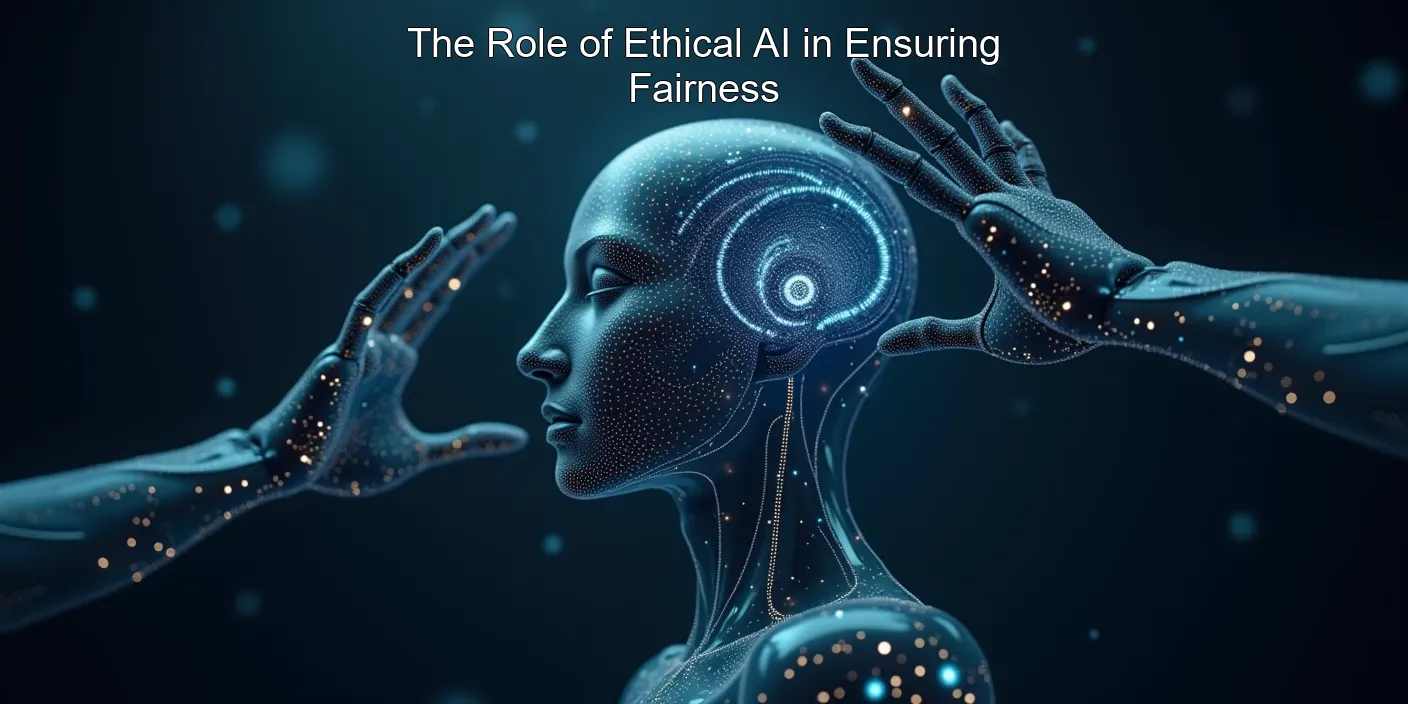
Ethical AI plays a crucial role in addressing fairness challenges. By incorporating ethical considerations into AI development, we can create systems that are not only powerful but also just and equitable.
“Ethical AI: Powering Fairness and Equity in Technology”
Ethical AI Principle | Impact on Fairness |
---|---|
Transparency | Enables scrutiny and correction of biases |
Accountability | Ensures responsibility for AI decisions |
Privacy | Protects individual rights and prevents discrimination |
Implementing ethical AI principles can lead to:
- Reduced algorithmic bias in decision-making processes
- Increased trust in AI systems among users and stakeholders
- Better alignment of AI outcomes with human values and societal norms
- Enhanced ability to detect and correct unfair AI behaviors
“Ethical AI is not just about following rules; it’s about creating AI systems that genuinely benefit humanity and promote fairness for all.” – Timnit Gebru, AI ethics researcher
Frequently Asked Questions about Ethical AI and Fairness
Q: How does ethical AI contribute to fairness?
A: Ethical AI frameworks provide guidelines for developing AI systems that respect human rights, promote equality, and avoid discrimination.
Q: Can AI ever be truly unbiased?
A: While perfect unbiased AI may be challenging to achieve, continuous efforts in ethical AI development can significantly reduce biases and improve fairness.
Q: What are some examples of ethical AI in practice?
A: Examples include AI-powered hiring tools that remove gender bias, facial recognition systems designed to work equally well across all ethnicities, and transparent AI decision-making in financial services.
Steps to Implement Ethical AI for Fairness
- Establish clear ethical guidelines for AI development
- Conduct regular ethical impact assessments
- Involve diverse stakeholders in the AI design process
- Implement robust testing for bias and fairness
- Provide mechanisms for human oversight and intervention
A report by Gartner predicts that by 2023, all personnel hired for AI development and training will have to demonstrate expertise in responsible AI, underscoring the growing importance of ethical considerations in AI.
The Future of Fair AI: Innovations and Challenges
As we look to the future, AI Innovations continue to push the boundaries of what’s possible. However, ensuring fairness remains a critical challenge that requires ongoing attention and innovation.
Future Trend | Potential Impact on Fairness |
---|---|
Federated Learning | Improved privacy and reduced data bias |
Quantum AI | Enhanced ability to process complex fairness constraints |
AI Governance Frameworks | Standardized approaches to ensuring AI fairness |
Emerging challenges in ensuring fair AI include:
- Balancing fairness with other AI objectives like efficiency and accuracy
- Addressing cross-cultural differences in fairness perceptions
- Managing the increasing complexity of AI systems
- Keeping pace with rapidly evolving AI technologies
“The future of AI is not just about making systems smarter, but making them fairer and more aligned with human values.” – Stuart Russell, AI researcher and author of “Human Compatible”
Frequently Asked Questions about the Future of Fair AI
Q: How will AI fairness evolve in the coming years?
A: Future developments are likely to focus on more sophisticated fairness metrics, advanced bias detection techniques, and AI systems that can self-correct for fairness.
Q: What role will regulation play in ensuring AI fairness?
A: Regulations are expected to become more comprehensive, potentially mandating fairness assessments
Challenges in Ensuring Fairness in AI Systems: Navigating the Ethical Landscape
Key Aspect | Challenge |
---|---|
Data Bias | Ensuring representative datasets |
Algorithm Design | Mitigating unintended discrimination |
Transparency | Explaining AI decision-making processes |
As artificial intelligence continues to shape our world, ensuring fairness in AI systems has become a critical challenge. Let’s explore the multifaceted issues surrounding this topic:
- Inherent biases in training data
- Complexity of defining “fairness” across diverse contexts
- Balancing accuracy with equitable outcomes
- Addressing historical inequalities in AI applications
“The biggest challenge in creating fair AI systems is not technical, but human. We must critically examine our own biases and societal structures to build truly equitable AI.” – Dr. Timnit Gebru, AI ethics researcher
Frequently Asked Questions
Q: What is algorithmic bias in AI?
A: Algorithmic bias refers to systematic errors in AI systems that can lead to unfair outcomes for certain groups, often based on race, gender, or socioeconomic status.
Q: How can we measure fairness in AI?
A: Fairness in AI can be measured through various metrics, including demographic parity, equal opportunity, and individual fairness, each addressing different aspects of equitable treatment.
Q: What role does transparency play in ensuring AI fairness?
A: Transparency is crucial for understanding how AI systems make decisions, allowing for scrutiny and correction of potential biases in the decision-making process.
Steps to Promote Fairness in AI Development
- Conduct thorough bias audits of training data
- Implement diverse and inclusive development teams
- Regularly test AI systems for fairness across different demographics
- Establish clear guidelines for ethical AI development
- Engage with affected communities for feedback and insights
According to a recent study by the AI Now Institute, only 15% of AI research papers explicitly consider fairness and ethical implications, highlighting the need for increased focus on these critical issues.
The Role of Regulation in Promoting AI Fairness
Regulatory Approach | Potential Impact |
---|---|
Mandatory Fairness Audits | Increased accountability |
Transparency Requirements | Enhanced public trust |
Ethical Guidelines | Standardized fairness practices |
Regulatory frameworks play a crucial role in addressing Ethical AI challenges. Let’s examine the current landscape:
- EU’s proposed AI Act focusing on high-risk AI applications
- US efforts to develop AI Bill of Rights
- Industry-led initiatives for self-regulation
- Global collaboration on AI governance standards
“Effective regulation of AI fairness requires a delicate balance between innovation and protection, ensuring that AI benefits all of society.” – Margrethe Vestager, Executive Vice President of the European Commission
Q: Can AI ever be truly fair?
A: While perfect fairness may be challenging to achieve, continuous improvement and vigilance can significantly enhance AI fairness over time.
Q: How does AI fairness impact everyday life?
A: AI fairness affects various aspects of AI in Everyday Life, from loan approvals to job recruitment, making it crucial for ensuring equal opportunities.
Q: What are the consequences of unfair AI systems?
A: Unfair AI systems can perpetuate and amplify societal biases, leading to discrimination and unequal access to resources and opportunities.
- Prioritize diversity in AI development teams
- Implement robust testing frameworks for bias detection
- Ensure transparency in AI decision-making processes
- Continuously update and refine AI models to address emerging biases
- Engage in ongoing dialogue with ethicists and affected communities
A survey by Gartner predicts that by 2023, all personnel hired for AI development and training will have to demonstrate expertise in responsible AI, underscoring the growing importance of fairness in AI systems.
The Future of AI Fairness: Innovations and Challenges
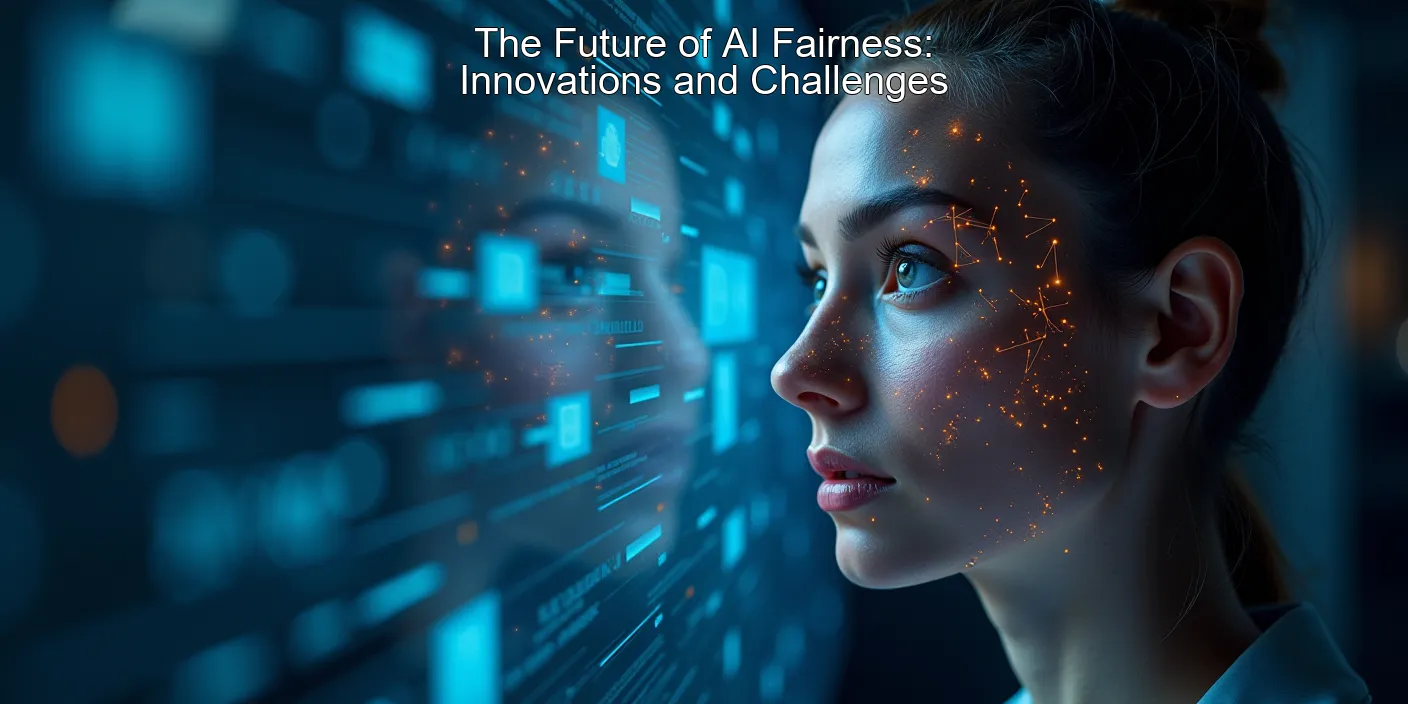
Innovation Area | Potential Impact |
---|---|
Explainable AI | Enhanced transparency |
Federated Learning | Improved data privacy |
AI Fairness Tools | Automated bias detection |
As we look to the future, several AI Innovations are poised to address fairness challenges:
- Advanced bias mitigation algorithms
- AI-powered fairness auditing tools
- Ethical AI frameworks integrated into development processes
- Cross-disciplinary approaches combining AI with social sciences
“The next frontier in AI fairness is not just about avoiding harm, but actively promoting equity and social good through intelligent systems.” – Fei-Fei Li, Co-Director of Stanford’s Human-Centered AI Institute
Q: How will AI fairness impact future technologies?
A: AI fairness will be crucial in shaping trustworthy and ethical AI in Entertainment and other industries, ensuring responsible innovation.
Q: What skills will be essential for future AI ethicists?
A: Future AI ethicists will need a combination of technical AI knowledge, ethical reasoning, and strong communication skills to navigate complex fairness issues.
Q: How can individuals contribute to AI fairness?
A: Individuals can contribute by staying informed, advocating for ethical AI practices, and participating in public discussions on AI governance.
Key Considerations for the Future of AI Fairness
- Developing global standards for AI fairness assessment
- Integrating fairness considerations into AI education curricula
- Encouraging interdisciplinary collaboration in AI ethics research
- Promoting public awareness and engagement in AI fairness issues
- Balancing innovation with ethical considerations in AI development
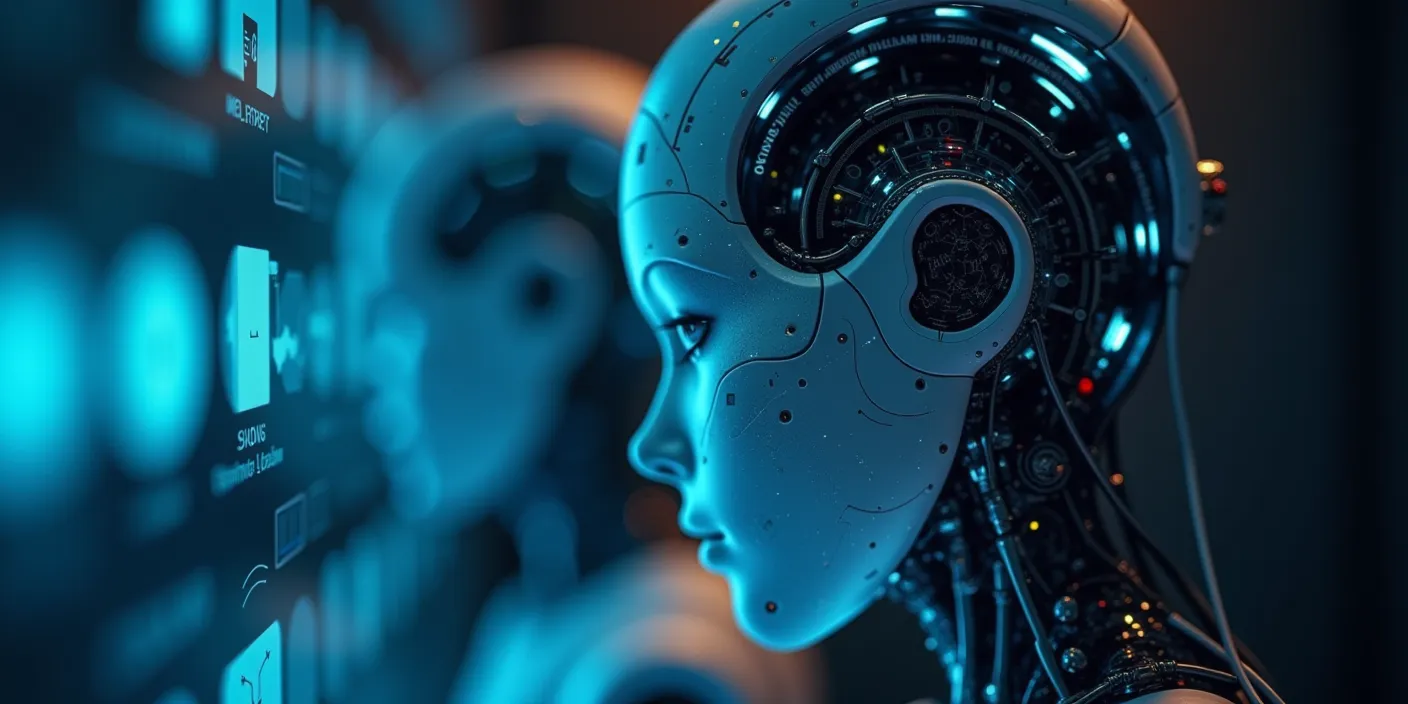
According to the World Economic Forum, addressing AI fairness challenges could unlock $5 trillion